- Home
- News
- Recent News
- Accelerating the Development of Low-Cost Rapid Diagnostics
Accelerating the Development of Low-Cost Rapid Diagnostics for Infectious Disease
Infectious diseases are a global health threat that is worsening, where major outbreaks are occurring more frequently.
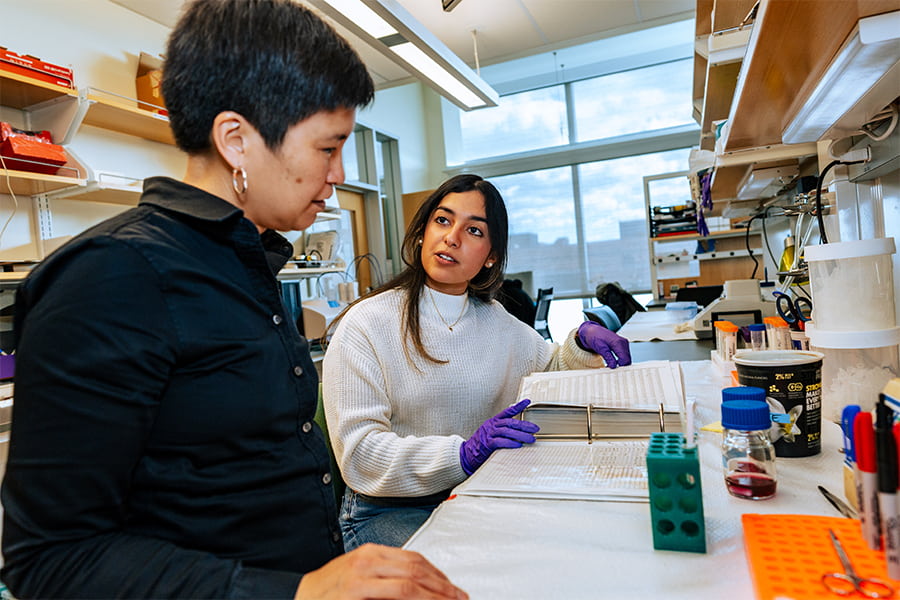
Infectious diseases are a global health threat that is worsening, where major outbreaks are occurring more frequently. As we saw with COVID-19, outbreaks are getting larger in scale, with far-reaching catastrophic effects. One critical tool for infectious disease is the diagnostic test, which enables decision-making for quarantining, patient treatment, and disease surveillance. While diagnostics have many forms, rapid tests that can be used at point of care have revolutionized global health. This is the format of the over-the-counter pregnancy tests and home COVID-19 tests. A biological fluid like a nasal swab is added to the paper test. If two red lines appear, the result is positive; one line, negative. Paper diagnostics are low cost, fully self-contained, and do not require power or specialized instruments. Consequently, they can be distributed on a massive scale, which is unfeasible for other diagnostics such as polymer chain reaction (PCR) tests.
However, current approaches for making paper diagnostics have several bottlenecks. They rely on antibodies specific for the virus, and generating antibodies must be produced in animals and then screened for those that bind to the target. The process is expensive and time-consuming, and significantly delays diagnostic distribution. Unfortunately, this limits our ability to respond quickly to diseases when they first emerge, the period when curbing its spread is most critical.
To address this problem, we are devising ways to get paper diagnostics on the ground sooner. First, to circumvent the generation of new antibodies every time an outbreak occurs, we are exploring a short cut and repurposing currently available antibodies that tend to bind off target. We demonstrated this by using antibodies intended for dengue and Zika viruses to detect yellow fever. We also used different-colored nanoparticles, so the test produced a range of colors instead of just red, and then used machine learning to train the system. By doing so, we could hack a commercial dengue diagnostic and convert it to a yellow fever test without any yellow fever antibodies.
Optimization of diagnostic performance is usually by trial and error, so we are also using machine learning to accelerate test development. We demonstrated this on a SARS-CoV-2 antibody test, which can distinguish whether a patient was infected vs. vaccinated based on the colorimetric pattern.
Finally, we are extending these strategies to design paper tests to detect unknown targets. When a new disease emerges, the only way to identify it is by DNA sequencing, which is slow, and samples must be sent to a centralized lab. To achieve this with paper tests, we use pattern recognition of the test lines to pick up disease targets that have not been identified before. We have demonstrated this on variants of SARS-CoV-2, where we used antibodies for the alpha variant to pick up unknown Omicron ones.
All of these strategies could be used in the field as they do not require instrumentation other than a mobile phone camera. Moreover, these strategies can be dispersed in communities to teach them how to make tests themselves using reagents that are on hand. Democratization of test production could make it more accessible and aid in curtailing outbreaks when they newly emerge.
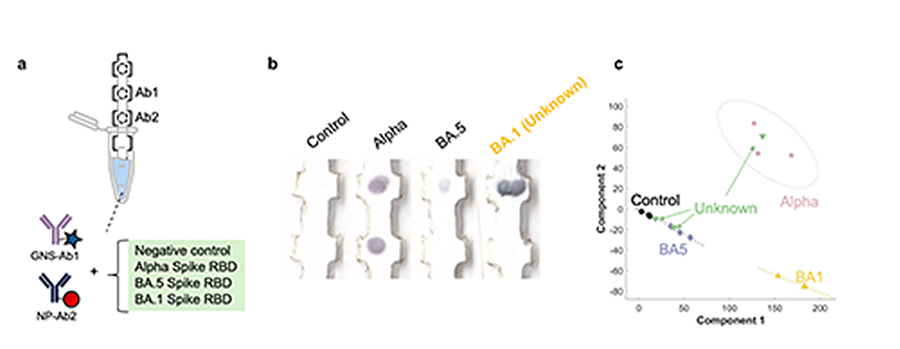
Paper test that can detect a variant of SARS-CoV-2 that it has not encountered before using antibodies only for the alpha variant. a) Schematic of the test that uses two different-colored nanoparticles. b) Images of test strips when run with different variants of SARS-CoV-2, which result in different-colored signals at two test areas. The test produces a signal when run with BA.1., a variant it has not seen before. c) Data analysis of the signal by principal component analysis shows that the new variant clusters separately from the other variants. Image By: Kimberly Hamad-Schifferli
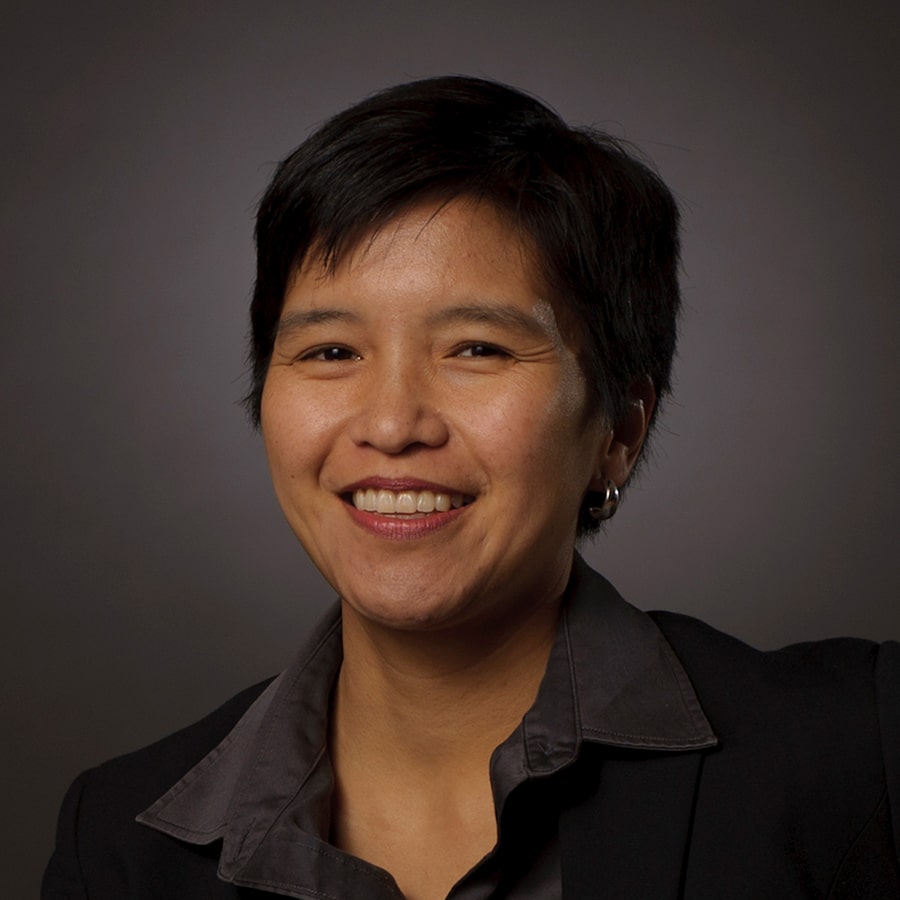
Professor of Engineering