- Home
- News
- Recent News
- Physics: New Approaches for AI Research
Physics: New Approaches for AI Research
A conversation about research in Physics and AI at UMass Boston
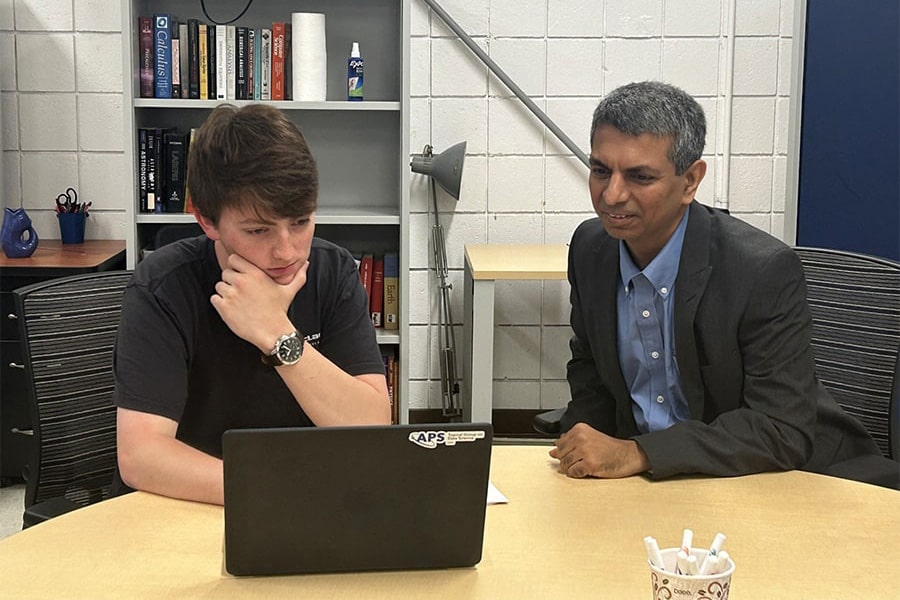
Jacob Adamczyk (JA): To begin, can you summarize the exciting and innovative research happening in the Department of Physics?
Rahul Kulkarni (RK): The Department of Physics at UMass Boston currently has two broad areas of research focus: biological physics and quantum science and technology. The application of fundamental concepts and results from statistical physics informs our research in both these areas. A field of research that we are currently looking into is the application of statistical physics to a new frontier: artificial intelligence (AI) research. Some of the new ideas in this field are being developed in our department, which is particularly exciting as we have a unique opportunity to forge new directions in current AI research.
JA: How did the connection with AI research develop within the Department of Physics?
RK: Typically, such a connection emerges from AI being used as a tool to study scientific datasets and classification problems in physics. However, we also saw the potential to follow a road less traveled: using physics insights to lead to new developments in AI research. More specifically, we saw reinforcement learning (RL) as a point for application of our ideas from studying nonequilibrium statistical physics. A surprising discovery we have made in this context is that diverse optimization problems in RL research can be mapped on to the physics of rare events.
JA: What is the physics of rare events, and what does it have to do with AI research?
RK: In RL, the goal is to learn how to optimize long-term rewards in problems involving sequential decision-making. If the agent employs an uninformed or random strategy for decision-making, high rewards will be obtained only very rarely. Take for example the game of chess, where a reward is obtained only at the end of a game for a win. Winning a game of chess by adopting a random strategy would clearly be a rare event against any reasonable opponent. The goal of reinforcement learning is to effectively discover policies that access such rare events. It turns out that in nonequilibrium statistical physics, approaches have been developed to characterize the “least unlikely of all unlikely ways” for such rare events to occur. Remarkably, these approaches also lead to optimal decision-making policies in reinforcement learning.
JA: What are the insights and successes that have emerged from this connection between AI and physics?
RK: We have used this bridge between nonequilibrium physics and AI research to develop new algorithms and theory for RL. Although we are still in the initial stages, we have solved some theoretical open problems in RL research and developed new algorithms for the field. There is still much more to explore, though. The exciting prospect is that these theoretical connections have the potential to speed up RL, making the algorithms more applicable to real-world scenarios.
Beyond theoretical and algorithmic advances, this connection also can provide a new understanding or intuition for AI algorithms from a physics perspective. At a more fundamental level, having a bridge between two previously unconnected disciplines means that deep insights, concepts, and approaches from one discipline can be potentially used to address currently challenging problems in the other discipline. For example, fundamental results from nonequilibrium statistical physics can now be used to derive new relationships between quantities of interest in AI research. On the flip side, novel approaches from AI research can now be used to address challenging problems of current interest such as quantum control and quantum circuit optimization.
JA: Both AI and quantum are seen as drivers of the next "Industrial Revolution." What unique contributions does the physics department bring to the table?
RK: The research done across the department is well-poised to contribute to research at the intersection of quantum physics and AI. Across the department, tools from statistical physics are used in a variety of applications (quantum systems, computational physics, nonequilibrium systems) and can thus contribute further to the bridge we have been discussing. Another exciting avenue that is ripe for exploration is the extension of ideas involving RL to the quantum domain, which many of our faculty have experience with. At the quantum foundations level, the department has started looking into whether AI approaches such as RL can be used to learn the fundamental rules of quantum mechanics. Our faculty are also looking into the extension of generative AI to the quantum domain and their connections to information-theoretic extensions of fundamental results in quantum statistical mechanics.
JA: Do we have plans to make changes to the curriculum to emphasize new tracks or courses related to these interdisciplinary connections?
RK: Absolutely. We already have an undergraduate Quantum Information Certificate (QIC) established, and one of our top priorities is building on this to develop a graduate version of the QIC. The graduate version will include modules on quantum machine learning and course material that integrates AI approaches with current advances in quantum information science. Initially, we plan to add AI-based modules to our existing courses, and we also want to develop a new class focusing on the intersection of AI research and physics. Eventually, the plan is to develop an AI and physics track that will build on some of the research being developed in the department. The goal would be to better prepare our students to be effective contributors to the modern workforce by developing expertise using AI tools, with a perspective that is guided by research in physics. As a matter of fact, the informal course that you have developed on physics and deep learning is an important first step in this direction, and we are looking into ways of offering the course more formally. Overall, the efforts in this direction are well-aligned with the University’s Strategic Priorities, including “Accelerating Interdisciplinary Scholarship” and “Education for the Future.“
JA: What might you say to a prospective student who is considering joining our department in light of these interdisciplinary connections?
RK: The UMass Boston Department of Physics has a unique offering of cutting-edge research directions. We currently have and are further developing specific tracks that will prepare you for a successful career in quantum information science, biophysics, and/or AI. The research within our department is also very interdisciplinary in its nature, allowing for collaborations with other fields such as computer science and biology.
Furthermore, there is a strong tradition of undergraduate research, with the department having nominated three Goldwater Scholars over the past five years. The American Physical Society (APS) has recognized our mentorship of undergraduate research with an award after one of our undergraduate students (Joseph Farah) was awarded the highest APS award for undergraduate research: the Leroy Apker Award.
Our graduate students are carrying out amazing research on a range of topics from quantum foundations and the physics of AI to cancer biophysics and soft matter physics. As part of their graduate training, students will benefit from hands-on experience with our labs as well as high-performance computing facilities. The interdisciplinary connections that we have discussed are being developed as we speak by graduate students in the department; this opens exciting possibilities for incoming students. The department is welcoming and supportive, and the faculty and existing students are eager to work with new students interested in exploring new frontiers.
JA: Finally, what are some of the open questions at the intersection of physics and AI that you're most excited to address next?
RK: Indeed, there are many exciting and open questions at this intersection. Currently, research in AI is in an exponential growth phase with new ideas and related research questions arising much faster than solutions. Remarkably, some of the answers to the questions being posed can be obtained from old insights in physics and mathematics research. For example, generative AI is one of the biggest drivers of the current excitement in AI. The original motivation for generative AI came from insights from physics-based models (diffusion models). One of the most promising ideas for current developments in this area harks back to an idea discussed by Schrödinger in the 1930s: the eponymous Schrödinger bridge.
In 1931, Schrödinger presented a paper to the Prussian Academy of Science entitled "On the reversal of natural laws" in which he considered the evolution of N independent Brownian particles. In the limit of large N, the evolution of the distribution of Brownian particles can be predicted using the Fokker–Planck equation. However, for finite N, the observed distribution can differ significantly from the predicted distribution. As N increases, such deviations from the predicted distribution are rare events. Given that such a rare event has been observed, Schrödinger posed the following question: What is the least unlikely way this rare event could have taken place? Schrödinger went on to propose a solution to this problem by deriving a set of equations that are now referred to as the "Schrödinger bridge." It was only decades later that it was realized that Schrödinger had posed and studied the problem of large deviations of the empirical distribution of Brownian particles. Recent research has further explored connections of the Schrödinger bridge to related fields of research such as optimal transport and generative AI. Given the mapping we have established between large deviation theory and RL, it appears that RL approaches can now be applied to Schrödinger bridge problems and generative AI, opening up exciting new avenues for future research.
Apart from this representative example, there are a host of interdisciplinary research projects that can now be approached using this mix of modern tools and older insights. Areas the department is planning to explore include applications to quantum sensing and control, AI approaches for quantum computing, and new methods for studying nonequilibrium systems. Beyond these exciting research directions, we also look forward to developing collaborations with neighboring institutions. We are currently collaborating with the Institute of Artificial Intelligence and Fundamental Interactions (IAIFI) at MIT on a project that has recently been funded by the National Science Foundation. The research focus of this partnership is on the development of novel RL approaches for the analysis of quantum systems. We plan on building on these ties to train the next generation of graduate students to carry out innovative research at the interface of physics and AI.
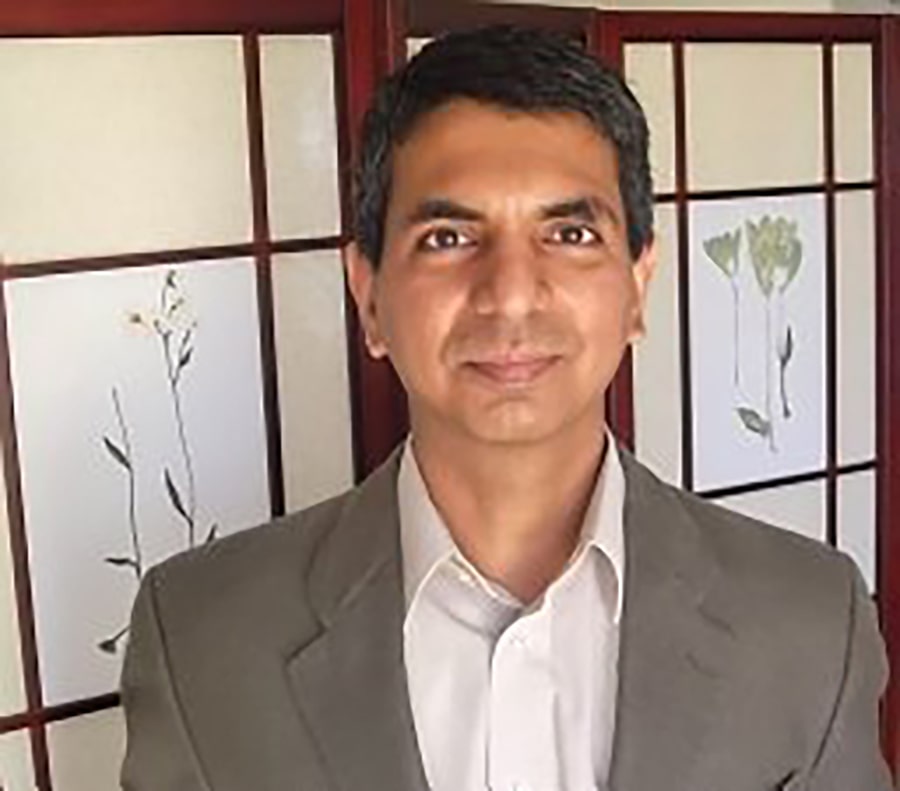
Department Chairman, Physics
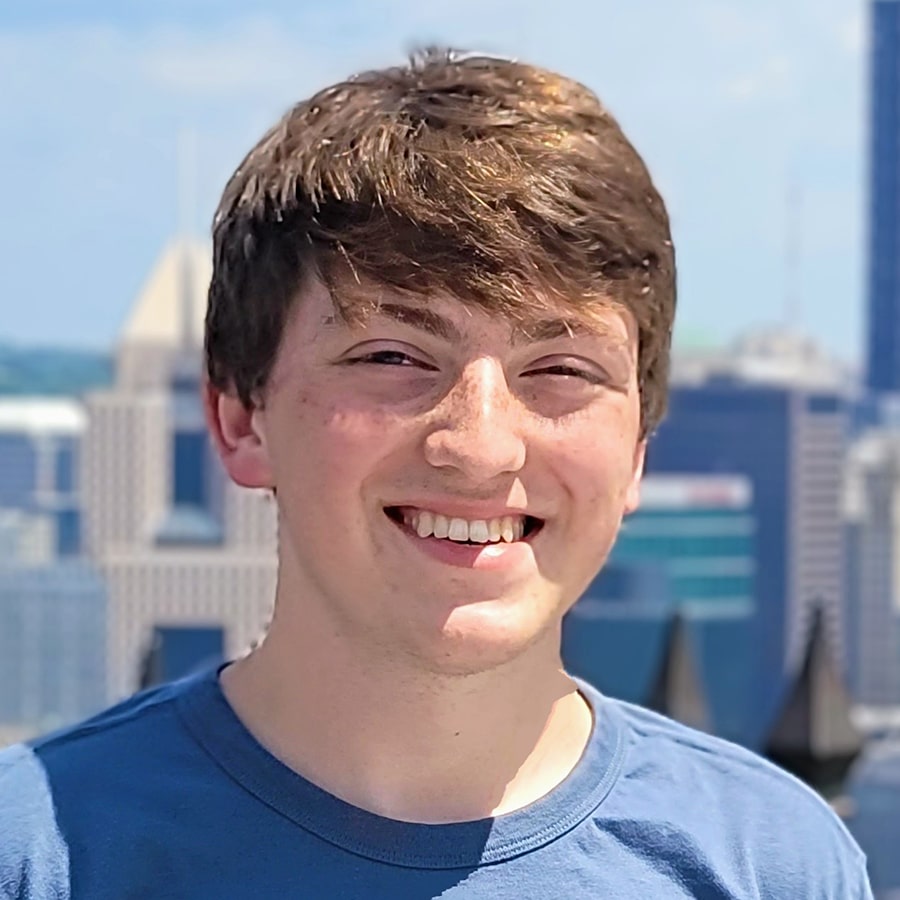
Physics